Natural language generation (NLG) is a critical area in AI, enabling applications such as machine translation (MT), language modeling (LM), summarization, and more. Recent advancements in large language models (LLMs) like GPT-4, BLOOM, and LLaMA have revolutionized how we interact with AI, using stochastic decoding to generate fluent and diverse text. However, evaluating the reliability of generated text remains a challenge, especially when applying pre-trained models to new, potentially divergent datasets, raising concerns about the generation of erroneous or misleading content. In this context, conformal prediction, a statistical method providing calibrated prediction sets with coverage guarantees, emerges as a promising solution. Yet, its application in NLG is not straightforward due to the conditional generation process, which violates the independence and identical distribution (i.i.d.) assumption central to conformal prediction.
To address this, the study draws on advancements in nearest-neighbor language modeling and machine translation. They propose dynamically generating calibration sets during inference to uphold statistical guarantees. Before delving into the method, it’s essential to grasp two key concepts: Conformal Prediction, known for its statistical coverage guarantees, and Non-exchangeable Conformal Prediction, which tackles the miscalibration caused by distributional drifts in non-i.i.d. scenarios by assigning relevance-weighted calibration data points.
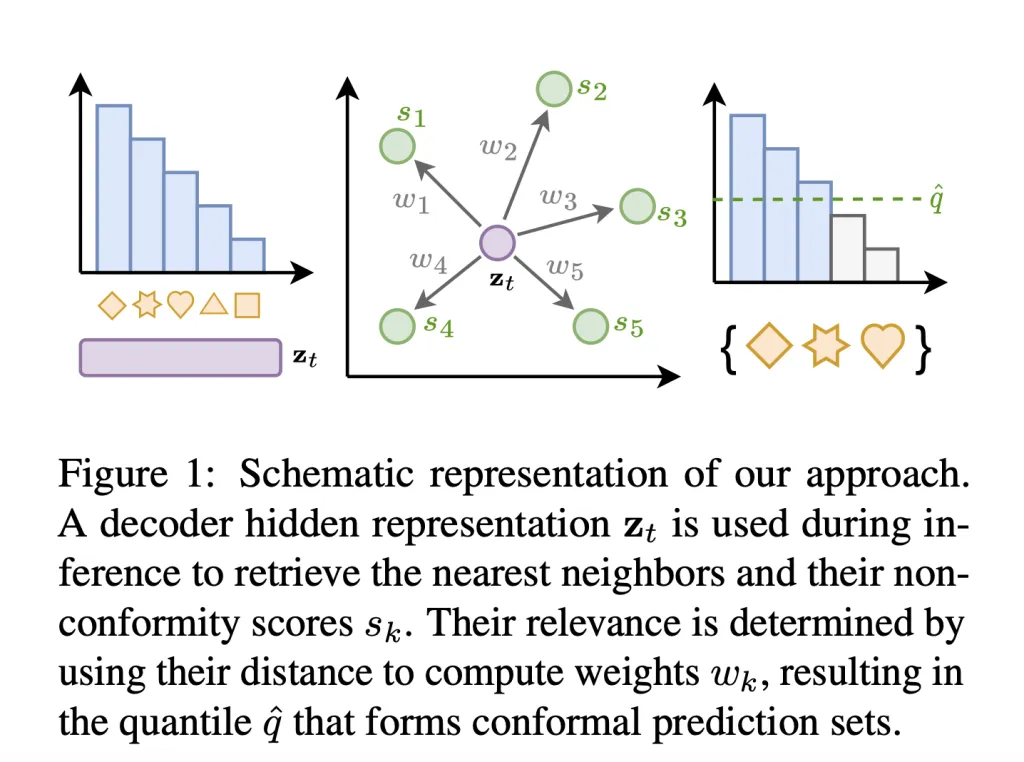
The method (shown in Figure 1), Non-exchangeable Conformal Language Generation through Nearest Neighbor, synthesizes the non-exchangeable approach with k-NN search-augmented neural models. It aims to generate calibrated prediction sets during model inference by only considering the most relevant data points from the calibration set. This is done by extracting decoder activations and conformity sources from a dataset of sequences and corresponding gold tokens, storing them for efficient k-NN search using FAISS. During inference, the decoder hidden state queries the datastore for the K nearest neighbors and their conformity scores, calculating weights based on the squared l2 distance. This approach contrasts with previous methods that produced overly broad prediction sets, highlighting its precision in generating relevant and statistically backed prediction sets.
Adaptive Prediction Sets play a crucial role, offering a more nuanced non-conformity score that accounts for language’s diverse nature. This approach encompasses a broader range of plausible continuations for challenging inputs, providing wider prediction sets where necessary.
Experiments in language modeling and machine translation, using models like M2M100 and OPT on datasets like WMT2022 and OpenWebText, showcase the method’s effectiveness. The use of FAISS for the datastore demonstrates the proposed method’s successful application, balancing high coverage with minimal prediction set sizes. The method’s ability to maintain coverage under distributional shifts is particularly notable, proving its robustness even with increasing noise variance.
In evaluating generation quality, the method does not compromise and, in some cases, even enhances the quality of generated content. It excels in generating statistically sound prediction sets while preserving or improving generation quality across different tasks.
In conclusion, this method marks a significant advance in conformal prediction application to NLG, adeptly handling the challenges of non-exchangeable data and maintaining desired coverage with smaller prediction sets. It not only offers theoretical guarantees about coverage but also demonstrates practical effectiveness in generation tasks. However, it’s crucial to recognize its limitations, such as potential issues with different dataset shifts and computational efficiency. Moreover, while conformal prediction provides a strong basis for reliability, it’s important to approach its application cautiously, especially in sensitive scenarios where distributional shifts are at work or when looking at specific subpopulations.
Check out the Paper. All credit for this research goes to the researchers of this project. Also, don’t forget to follow us on Twitter and Google News. Join our 37k+ ML SubReddit, 41k+ Facebook Community, Discord Channel, and LinkedIn Group.
If you like our work, you will love our newsletter..
Don’t Forget to join our Telegram Channel
The post This AI Paper Unveils a New Method for Statistically-Guaranteed Text Generation Using Non-Exchangeable Conformal Prediction appeared first on MarkTechPost.
#AIShorts #Applications #ArtificialIntelligence #EditorsPick #LanguageModel #LargeLanguageModel #Staff #TechNews #Technology #Uncategorized [Source: AI Techpark]