As AI tools find their way into just about every aspect of modern life, the question remains as to how society can ensure this technology is developed and used responsibly. The efficiency of AI offers many advantages, but it could also introduce new problems.
Issues specifically dealing with privacy, data governance, transparency, explainability, fairness, security, and safety all fall under the purview of responsible AI.
The responsible implementation of AI is so important that Stanford University’s Institute for Human-Centered AI (HAI) spent an entire chapter of its AI Index Report on the subject. This report is the seventh-annual deep dive into a variety of important aspects of the growing field of AI, as well as how these topics impact human users.
To learn more about responsible AI, I interviewed Anka Reuel. A Ph.D. student at Stanford University and a Stanford HAI Graduate Fellow, Reuel acted as the lead for the responsible AI chapter. Her insight will shed light on the complex challenges that await the future of AI.
A Lack of Standardization
While there was much to discuss in the full AI Index Report, a specific aspect of responsible AI that must be addressed soon is the lack of standardization in responsible AI reporting. Reuel said that the major challenge in evaluating AI systems – particularly foundation models – has to do with how versatile these tools are. Modern AI systems are not designed for a single task, but rather to adapt to a variety of contexts and objectives.
“As such, it becomes difficult to evaluate them in their entirety since creating evaluations for every potential use case is impractical,” Reuel said. “Ideally, we would want robust evaluations that assess the inherent abilities of these models, but the current predominant prompt-based evaluations fall short in this regard.”
While Reuel conceded that non-generative, task-specific AI systems have more straightforward evaluation benchmarks – as there is a single task to assess them against – even these cases find their benchmarks becoming saturated quickly, considering how fast the field of AI is growing.
Additionally, Reuel pointed out that simply deciding on certain benchmarks can prove problematic.
“Another issue arises when public benchmarks inadvertently become part of the training data for these models, as they re trained on vast portions of the internet,” Reuel said. “This can effectively contaminate the model, akin to providing high school students with the actual test questions before their final exam. They may perform better, but it doesn t necessarily reflect a deeper understanding of the concepts being evaluated.”
Another important aspect that must be addressed in the testing of responsibility with AI systems is that we currently lack comprehensive red-teaming methods that can properly address the broad variety of prompts that can elicit unwanted behavior. More specifically, she mentions that diversifying red-teaming efforts will be crucial, both in terms of the people involved and the tools that are used.
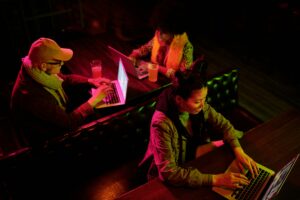
Diversity within red-teaming groups will be absolutely crucial in discovering issues within AI models.
“For instance, having a red-teaming team solely comprised of white, male developers is not going to cut it; a more diverse team has a significantly higher chance of catching issues with the model,” Reuel said. “Additionally, employing a combination of manual/human red-teaming, automated red-teaming, and language-model-based red-teaming can help uncover a wider range of vulnerabilities.”
This is a valuable point, as the report also discussed a survey that found most companies have only mitigated a portion of the AI risks that they currently face. The report also found that there was a 32.2% increase in AI misuse incidents since 2022 and a more than twentyfold increase in incidents since 2013, specifically citing the use of AI tools to create sexually explicit deepfakes of Taylor Swift.
Clearly, more needs to be done to ensure that AI tools are both used and deployed in a responsible manner.
Legal Questions Remain
On top of the general ethical discussion surrounding responsible AI, there are also political and legal issues to address. The responsible AI chapter has an entire section devoted to AI and elections, with a specific focus on the generation of disinformation. The report correctly mentions that disinformation campaigns can undermine trust in democratic institutions and manipulate public opinion. What’s more, deepfake tools have significantly improved since the 2020 U.S. election.
Additionally, the report mentions that automated dissemination tactics have grown rapidly in recent years. While many have been concerned with AI-generated content, it would appear that bad actors have now figured out how to automate the entire generation and dissemination pipeline. A developer called Nea Paw in the report created something called CounterCloud as an experiment in the automated generation and dissemination of false content.
The system scraped the internet for timely articles to decide which content it should target with counter-articles. After writing the counter-articles, the content is attributed to a fake journalist and posted on the CounterCloud website. Then, a different AI system generates comments on the articles to falsify organic engagement before posting the articles as replies and comments on X. The entire setup for this misinformation system only costs about $400. Overcoming these new and frightening tools for misinformation will demand hard work from AI experts as well as regulation.
In a similar vein, the report also discussed the potential for AI systems to output copyrighted material. Reuel mentions that currently pending lawsuits will most likely lead the way to the solution to this problem.

Many legal cases will need to be settled before we can begin to understand what copyright and licensed data will look like in a world with AI.
“Depending on the outcomes of lawsuits like NYT vs. OpenAI this year, we may witness a significant shift towards models trained exclusively on licensed data,” Reuel said. “This would especially hold true if developers are found liable for copyright infringements or prohibited from using data without consent or licenses.”
That said, there is the specific concept of “copyright infringement” that must be addressed. As with most things during these early years of the AI revolution, one-definite definitions are beginning to blur and become more complex.
“A key question policymakers must address is: what constitutes copyright infringement in the context of foundation models?” Reuel said. “Is training on data without consent already an infringement? Or is it only when models output training data verbatim? How much must output deviate from the original input to avoid infringement? And where lies the line between infringement and creativity? These are complex questions that will require a dialogue among all impacted stakeholders.”
One of the ways forward here will be to encourage and outright demand transparency from the creators of these AI models. Specifically, in October 2023, Stanford, Princeton, and MIT researchers released the Foundation Model Transparency Index (FMTI). Designed around 100 transparency indicators, the FMTI is meant to assess the transparency of foundation models like GPT-4 and Llamma 2.
As the AI Index Report indicates, the FMTI shows a severe lack of transparency from AI developers, which hinders the public’s ability to understand each model s responsibility and safety. Again, it would seem that the world is waiting for the legal system to work out these issues.
“The lack of transparency from developers likely partly stems from unresolved legal questions surrounding issues like copyright infringement,” Reuel said. “Establishing a clear legal framework that provides certainty may contribute to greater transparency from foundation model developers. Conversely, if organizations utilizing these models start demanding more transparency and prioritizing foundation model developers that are more transparent, we may witness a shift even without legally binding obligations.”
As AI capabilities rapidly expand into various facets of society, ensuring its responsible development and implementation grows increasingly crucial. We must collectively prioritize transparency from AI creators, establish robust evaluation standards across diverse perspectives, and develop legal frameworks that safeguard privacy, fairness, and security. Only through coordinated efforts involving technologists, policymakers, and the public can we fully harness AI s potential while mitigating risks.
#AI/ML/DL #Slider:FrontPage #AIinlegal #AIIndexReport #responsibleAI #Stanford #StanfordHAI [Source: EnterpriseAI]